Unveiling Potential: The Role of Data Enrichment in Analysis
Unveiling Potential: The Role of Data Enrichment in Analysis
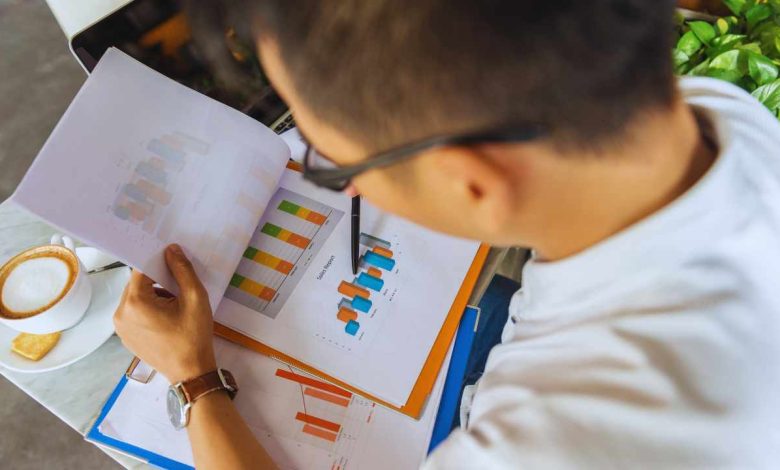
In today’s data-driven world, businesses are constantly seeking ways to extract valuable insights from the vast amounts of data at their disposal. Data enrichment has emerged as a crucial tool in this endeavor, offering a means to enhance the quality and depth of available data for analysis. This article explores the significance of data enrichment in analysis and its role in unlocking hidden potential.
Understanding Data Enrichment
What is Data Enrichment?
Data enrichment is the process of enhancing raw data by adding additional information to it. This additional information can include demographic data, geographic data, social media profiles, purchasing behavior, and more. By enriching raw data, organizations can gain a more comprehensive understanding of their customers, products, and markets.
How Does Data Enrichment Work?
Data enrichment typically involves accessing external data sources or using algorithms to fill in missing information or enhance existing data points. This can be done through various methods such as data appending, data cleansing, and data normalization. The goal is to enrich the data to make it more valuable and actionable for analysis.
The Benefits of Data Enrichment
Enhanced Data Accuracy
One of the primary benefits of data enrichment is improved data accuracy. By supplementing raw data with additional information, organizations can fill in gaps and correct inaccuracies, resulting in a more reliable dataset for analysis.
Improved Decision Making
With enriched data, organizations can make more informed decisions. By having access to a wealth of relevant information, decision-makers can gain deeper insights into customer behavior, market trends, and competitive landscapes, enabling them to make strategic decisions with confidence.
Better Customer Insights
Data enrichment enables organizations to gain a deeper understanding of their customers. By enriching customer data with demographic, psychographic, and behavioral information, businesses can create more targeted marketing campaigns, personalized customer experiences, and tailored product offerings.
Applications of Data Enrichment
Marketing and Sales
In marketing and sales, data enrichment plays a critical role in lead generation, customer segmentation, and personalized marketing efforts. By enriching customer data with information such as purchasing history, browsing behavior, and social media activity, businesses can create more targeted and effective marketing campaigns.
Customer Relationship Management (CRM)
Data enrichment is also valuable in customer relationship management (CRM). By enriching customer profiles with additional information such as contact details, company information, and social media profiles, businesses can better understand their customers’ needs and preferences, leading to improved customer engagement and loyalty.
Personalization and Targeting
Data enrichment enables businesses to deliver personalized experiences to their customers. By enriching customer data with information such as preferences, interests, and behavior patterns, organizations can tailor their products, services, and marketing messages to individual customers, increasing engagement and satisfaction.
Challenges and Considerations
Data Privacy and Security
One of the primary challenges of data enrichment is ensuring data privacy and security. As organizations collect and use large amounts of data from various sources, they must adhere to strict data protection regulations and implement robust security measures to safeguard sensitive information.
Integration Complexity
Another challenge of data enrichment is the complexity of integrating disparate data sources. Organizations often deal with data silos and incompatible data formats, making it challenging to integrate and enrich data effectively. Addressing these integration challenges requires careful planning and investment in advanced data integration technologies.
Best Practices for Implementing Data Enrichment
Define Clear Objectives
Before embarking on a data enrichment initiative, organizations should define clear objectives and goals. By understanding what they hope to achieve through data enrichment, businesses can tailor their strategies and focus their efforts on areas that will deliver the most significant impact.
Choose Reliable Data Sources
When enriching data, it’s essential to use reliable and trustworthy data sources. Organizations should carefully vet data providers and ensure that the data they use is accurate, up-to-date, and compliant with relevant regulations.
Regular Data Maintenance
Data enrichment is an ongoing process that requires regular maintenance and updates. Organizations should establish processes for monitoring data quality, identifying inaccuracies or inconsistencies, and updating enriched data to ensure its continued relevance and accuracy.
Real-World Examples
Case Study 1: Retail Industry
A leading retail chain used data enrichment to enhance its customer profiles with demographic and purchasing behavior data. By leveraging enriched customer data, the company was able to segment its customer base more effectively and personalize its marketing campaigns, resulting in increased sales and customer satisfaction.
Case Study 2: Healthcare Sector
A healthcare provider implemented data enrichment to improve patient outcomes and operational efficiency. By enriching patient records with medical history, treatment data, and diagnostic information, the organization was able to identify patterns and trends, streamline workflows, and deliver more personalized care to patients.
Future Trends in Data Enrichment
AI and Machine Learning Integration
The future of data enrichment lies in AI and machine learning technologies. These advanced analytics techniques can automate and optimize the data enrichment process, enabling organizations to extract insights more quickly and accurately from large and complex datasets.
Predictive Analytics
Another emerging trend in data enrichment is predictive analytics. By leveraging enriched data and advanced modeling techniques, organizations can anticipate future trends, behaviors, and outcomes, enabling them to make proactive decisions and stay ahead of the competition.
Conclusion
Data enrichment is a powerful tool for unlocking the potential hidden within vast datasets. By enhancing raw data with additional information, organizations can gain deeper insights, make more informed decisions, and drive better business outcomes. As data continues to proliferate and become more complex, the role of data enrichment in analysis will only become more critical in helping organizations stay competitive in the digital age.